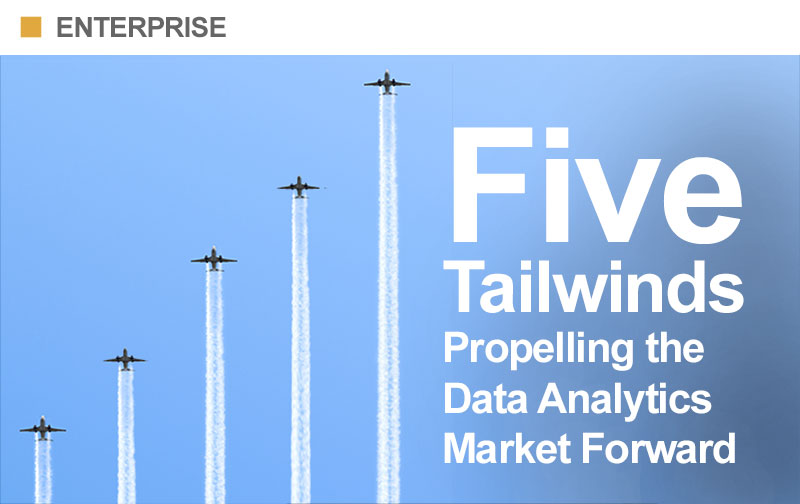
By Eric Lee, Principal, and Rich Ellinger, Partner, at Cota Capital
Data analytics is one of the largest and most exciting areas in the software industry. With a current market size of $119 billion, data analytics is nearly 3x larger than the ERP (enterprise resource planning) market and 6x larger than the application development market, in each case according to Gartner. Today, businesses large and small can harness the power of data analytics to gain insights, make real-time decisions, and drive growth.
With companies running billions of queries every day and processing exabytes of data annually, the scale of data-driven operations is jaw-dropping. And it’s just getting started, as the market is projected to double in size over the next four years, according to the Gartner Enterprise Infrastructure Software Forecast. With the rise of cloud-first players, data analytics is forecast to grow at 1.6x the rate of total IT spend, says Gartner.
Now that data analytics has become the decision-making backbone for enterprises worldwide, private capital has taken notice. Indeed, AI/ML and analytics are now two of the three top-funded cloud markets. Today, the top 50 private data analytics companies boast a collective valuation of over $100 billion, underscoring the tremendous opportunities in the market.
Furthermore, AI models are only as good as the data that they’re fed. Thus, it’s becoming increasingly apparent that companies can’t formulate an AI strategy without first establishing a well-defined data strategy. As technology continues its rapid advancement, data analytics will remain at the cutting edge of innovation, shaping the future in ways we cannot yet fully imagine.
Here are five key tailwinds that are propelling the market forward.
1: Surging volume of data
Data production is doubling every two years, with a 50-fold increase in data volume from 2010 to 2020, according to the Worldwide IDC Global DataSphere Forecast. Diverse data sources, including human, machine, and traditional data—as well as unstructured data like online video, audio, and images—contribute to this growth. Additionally, there are an increasing number of data sources such as sensors from connected devices or synthetic data to train neutral networks and machine learning models.
This abundance of data, and the speed at which it is being generated, has spurred a shift from batch processing to real-time analysis. Despite the complexity of handling unstructured data, the opportunities for businesses to gain valuable insights and make data-driven decisions are immense, offering significant potential for further innovation and growth.
2: Compute and storage improvements
The performance of compute systems per dollar invested has seen substantial improvements, doubling every 2.5 years. Businesses can now process vast amounts of data at a fraction of the cost compared to previous years. This has played a pivotal role in accelerating data analytics and making it more accessible to a broader range of organizations. Storage expenses have also plummeted, experiencing an 800-fold reduction since 2000. This cost reduction has been a game-changer for data analytics, as it allows companies to store and retain massive volumes of data that in the past would have been discarded due to cost constraints.
The shift toward cloud and hybrid infrastructure has also changed the game. By leveraging cloud-based solutions, companies can access more scalable and flexible analytics and storage capabilities. This enables them to efficiently manage higher data volumes and diverse data types. Additionally, thanks to improved compute and storage capabilities, businesses are better prepared to implement advanced analytics use cases, helping them uncover valuable patterns and trends that were previously hidden from them.
3: Emerging use cases
Needs have changed dramatically over the past 20 years. The market has transitioned from traditional descriptive analytics to modern predictive/prescriptive analytics and increasingly to emerging use cases. Descriptive analytics focuses on understanding past performance by analyzing historical data. Predictive/prescriptive analytics aims to forecast future outcomes based on historical data.
Emerging use cases are the next frontier, enabling real-time insights and decision-making within seconds or minutes. This includes data sharing, which integrates internal and external data for deeper insights. Additionally, the analysis of data stored across multiple cloud environments is growing in importance. Another key aspect of emerging use cases is the introduction of self-serve capabilities, which allow nontechnical staff to run queries and generate reports without relying on IT support. This empowers individuals throughout the organization to leverage data efficiently and make the best business decisions going forward.
4: The democratization of data
The democratization of data has led to increased market reach for analytics by opening up access to a wider range of users. Organizations are investing in both in-house and external data science training courses to enhance data literacy. Advanced analytics tools are being developed to abstract the complexity of coding, allowing lower-skilled users to access and utilize sophisticated analytical techniques.
The demand for data scientists is projected to grow significantly, with an estimated 36% increase from 2021 to 2031, surpassing the average growth rate for all other occupations. Fortunately, the availability of more accessible technology is lowering the barrier of entry to the data science field. Professionals from various backgrounds, not only computer science or statistics, can now leverage data to gain insights, ultimately contributing to a more data-literate and data-driven workforce.
5: New architectures that enable the data analytics stack
Advancements in technology architectures have profoundly impacted data analytics solutions and brought more sophisticated capabilities. Key advancements include cloud-based data platforms, real-time data processing, modular platforms, decoupled data access, domain-based architectures, and flexible data schemas.
For instance, modular architectures now allow businesses to choose and integrate the best tools for each specific task, instead of relying on monolithic systems. This flexibility fosters efficiency and adaptability within the data analytics stack. Additionally, employing flexible and extensible data schemas accommodates diverse data types and evolving business requirements. These components empower organizations to efficiently manage and analyze data, enabling informed decision-making and business insights.
Prospective effects
These tailwinds will only accelerate with the rise of AI, which is making data analytics faster, smarter, and more accessible. As the field of data analytics continues to advance, opportunities will abound for startups to innovate, disrupt, and shape the future of data-driven decision-making. Those who effectively harness the power of data analytics may gain unique insights into trends, opportunities, and market gaps that competitors may miss. This can translate into an edge over rivals for years to come.